Cataract Patients See Better Results with AI
AI Modeling Technique from the Automotive Industry Improves Eye Surgery Results
A cataract is an opacity that forms within the lens of the eye, causing blurred vision. If the opacity is very dense, blindness may result. Millions of people turn to cataract surgery each year to restore their vision. During the procedure, an ophthalmologist surgically removes the natural lens of the patient’s eye and replaces it with an artificial one called an intraocular lens (IOL). Cataract patients rely on their surgeon’s training and experience. They also depend on the surgeon to select the correct power IOL. For some special types of IOLs, the implantation of the correct power determines the procedure’s success.
“We would all like every patient to have a perfect result. Traditional mathematics really didn’t allow us to get the right answer as often as we would like.”
Some measurements of the human eye may vary significantly from person to person. These variations make it difficult to accurately predict the power of the artificial lens needed for an optimal postoperative result. Ophthalmologists have long relied on formulas based on traditional Gaussian optics to estimate the correct IOL power. The calculations utilize standard measurement techniques and a wide range of commonly used formulas. A result of ±0.50 diopters (D), a measure of lens power, is considered a successful surgical outcome. The calculations typically provide a 78% success rate within ±0.50 D. With an estimated 28 million people receiving cataract surgery worldwide each year, the success rate leaves many patients with a result that may be different than anticipated.
Cataract symptoms include color perception changes due to yellowing of the lens, blurred vision, and double vision or “ghosting.”
“Although refractive outcomes continue to improve, all of us in ophthalmology who do cataract surgery occasionally struggle with the outcomes. Of course, we would all like every patient to have a perfect result,” says Warren Hill, M.D., an ophthalmologist who has been performing cataract surgeries for 35 years. “Traditional mathematics didn’t allow us to get the right answer as often as we would like.”
Dr. Hill explains that ophthalmologists have been making strides toward more precise calculations over the last 40 years but describes these improvements as “painfully incremental.” Part of the problem, he says, is that not enough ophthalmologists have been thinking outside the box—an issue that doesn’t plague Hill. He is a trained artist, experienced writer, and well-practiced doctor. Hill is also a commercial pilot and a member of a close-formation military airplane demonstration team in his spare time. He constantly seeks new information.
Hill’s thirst for new information was particularly fruitful after a 10-year endeavor to better calculate individual cataract patients’ IOL power, regardless of their unique eye structure. Hill’s approach, which harnesses the power of artificial intelligence (AI), drove the ±0.50 D surgical success rate to 90%.
Lessons from the Automotive Industry
Hill realized that a completely novel way to calculate the lens power was needed. “Ophthalmologists know a lot about eye surgery, but almost none are trained as mathematicians or engineers,” he concedes. “You have to be willing to go outside of your specialty to solve long-standing problems in new ways.”
Hill knew MathWorks provided industrial-strength math tools and thought MATLAB® might be useful for modeling patient data. He partnered with engineers at MathWorks, one of whom was familiar with a similar modeling problem from the automotive industry: powertrain optimization. To optimize engine fuel efficiency and performance, automotive companies use response surface models such as Radial Basis Functions to relate engine control settings to engine responses and find the optimal combination of engine settings to store in the electronic engine controller.
Pete Maloney, a development engineer at MathWorks, became familiar with this approach through his previous automotive industry experience. When Hill approached the team at MathWorks with the goal of optimizing the optical power of intraocular lenses for cataract surgery patients, Maloney understood that the radial basis function (RBF) used to model complex engine behavior in automotive engine calibration optimization could also be applied to a problem like this.
“Completely new ideas in mathematics are relatively uncommon,” observes Hill, “but applying existing ideas in completely new ways is a very common approach to problem-solving.”
A predictive RBF network model must be trained with high-quality data. Existing data for eye measurements had previously been gathered using inconsistent and sometimes poorly validated methods. Hill knew that getting a good RBF model fit and prediction would require much higher-quality data than normally available.
Hill reached out to Doug Koch, M.D., a professor of ophthalmology at Baylor College of Medicine, and Li Wang, M.D., Ph.D., an associate professor of ophthalmology at Baylor College of Medicine, with experience in biomedical statistics. Together, they outlined the level of accuracy that a project such as this would require to be successful.
With appropriate methods and standards for data collection in place, Hill then sought to recruit the most experienced ophthalmologists he knew, enlisting trusted colleagues both in the United States and abroad. These ophthalmologists began collecting detailed measurements of patients’ eyes before cataract surgery using Haag-Streit’s highly accurate Lenstar biometer, along with the observed postoperative outcomes.
Based on 802 validated measurements and postoperative results, the initial effort tested the premise that an artificial intelligence model could accurately calculate the power of an intraocular lens to be placed inside the human eye. The predicted outcomes for this relatively small dataset easily met the criteria outlined by Dr. Wang. With that hurdle cleared, the project moved forward to create a completely new tool for ophthalmic surgeons to use to obtain improved accuracy for cataract surgery refractive outcomes.
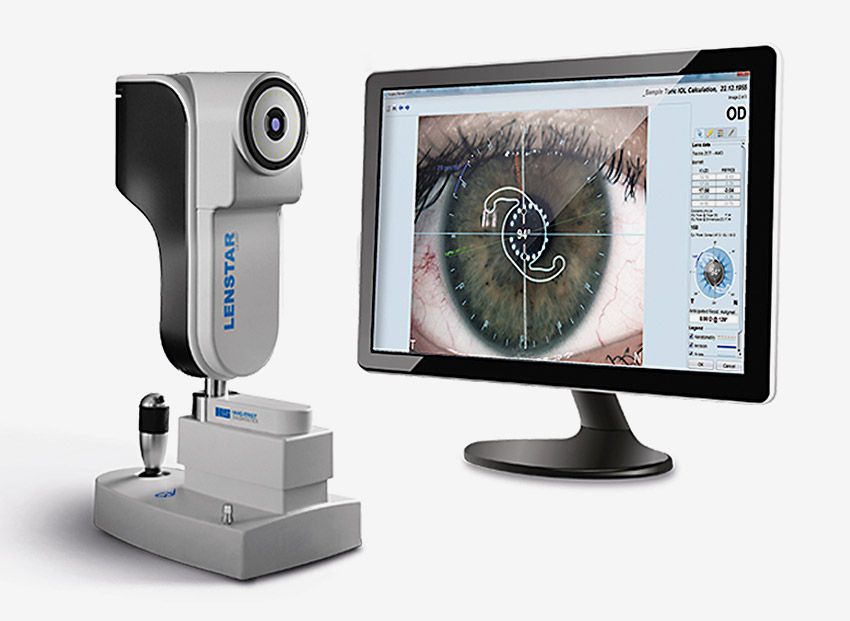
The Haag-Streit Lenstar 900 biometer uses the Hill IOL Calculator. (Image credit: Haag-Streit Diagnostics)
Seeing Clear Results
Next, MathWorks used a much larger dataset of approximately 3,400 surgical cases by surgeons in many countries around the world and fit an RBF model to this data. The team exported the model to Simulink®, a graphical environment for designing, simulating, and testing systems, and then generated code from the model and deploy it to the Lenstar device. The calculator is known as the Hill-RBF calculator. An online version of this calculator was also released simultaneously, so any ophthalmologist worldwide would have access.
“We’re on version three [of the Hill RBF calculator] and, you know, from my wheelchair at the nursing home I'll probably be working on version 48, just trying to make it better and better.”
The calculator, launched in 2016, was quickly adopted by the worldwide ophthalmic community. Upon seeing the calculator’s success, Hill’s team decided to refine the tool further. This involved reaching out to more ophthalmologists, training them on collecting the data, and creating a more inclusive and accurate AI model. In total, 44 investigators across 20 countries have participated. Surgeons who contributed their cases have all done so on a volunteer basis.
Using updated and refined versions of the calculator that incorporate a much larger dataset, surgeons are now seeing outcomes at a 90% ±0.50 D accuracy, compared with the 78% success rate of the more commonly used traditional and older Gaussian formulas. To put that in perspective, with approximately 28 million surgeries conducted worldwide annually, a 12% improvement in outcomes would result in 3.4 million additional surgical successes. For people with myopia, the calculator’s success rate is even higher.
“This has been a major advance in ophthalmology,” says Hill. “Calculating the power of IOLs in a consistently accurate manner is something we have been struggling with for 40 years, and, thanks to the work of a lot of people, we were able to dramatically increase the accuracy.”
As Hill attended conferences, presented multiple named lectureships on this topic, and spread word of the calculator around the world, the calculator steadily gained popularity. He has made this tool available online for anyone to use and at no charge from the very beginning.
The second version of the Hill RBF calculator, based on data from more than 12,000 eyes, was launched in 2018. It has become the second most popular calculator in North America for Lenstar users for estimating the power of intraocular lenses, and has been used in more than 2 million operations worldwide.
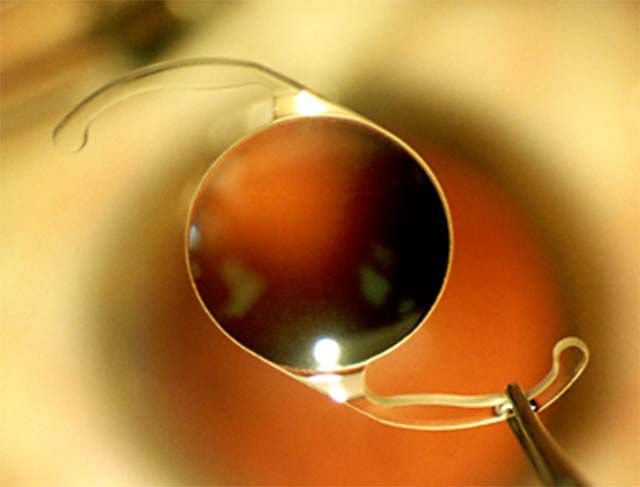
Single-piece acrylic IOL prior to its insertion into the eye. (Image credit: Warren Hill, M.D.)
The recently launched third version relies on data from an expanded number of eyes. Now, Hill’s team is working on a completely new version of the calculator, including data from new populations through collaboration with ophthalmologists from other countries, such as China. Eyes frequently show anatomic differences across populations, and optimizing an AI IOL power selection model specifically for these groups offers the possibility of improved calculation accuracy.
Another important outcome of the Hill RBF calculator is the ability to better predict the likelihood of successful surgery for individual patients. Embedded in the tool is what is known as a boundary model. This is an additional model (convex hull) of the outer boundaries of the training dataset. If a person’s eye varies too much from the training data, this boundary model will indicate that the RBF IOL prediction may not have sufficient data to determine the correct lens power.
“It’s the first time in eye surgery that anybody’s been able to be told, prior to surgery, the chances of having accuracy at a certain level,” explains Hill.
He says that working on this project has been very rewarding, especially in terms of feedback from ophthalmologists from around the world. “Many surgeons are now using this calculator exclusively and their outcomes are quite amazing,” says Hill.
Hill remembers his father once telling him that there is nothing more powerful than a good idea. He considers the use of AI for cataract surgery IOL power selection to be a once-in-a-lifetime good idea he is proud to share with the world.
“And we’re going to make it even better. We’re on version three and, you know, from my wheelchair at the nursing home I’ll probably be working on version 48, just trying to make it better and better.”
More Articles on AI
Read Other Stories
GREEN TECHNOLOGY
Unplugged: The New Way to Charge Electric Vehicles
Wirelessly Charging McLaren’s Fastest Road Car Ever
BIOTECH
When You Know the Answer, Deep Learning Can Determine the Question
Machine Learning Starts with a Biochip’s Function and Works Backward to Design Its Form
ROBOTICS / AUTONOMOUS SYSTEMS
Trusting Robots to Navigate New Spaces
New Algorithm Boosts Robustness of Robot Perception